ADM1F_SRT: Input/output sensitivity¶
Here we explore the relationships between inputs and outputs. In the ADM1F: Execution time
example we showed how to run the models with the perturbed input values from influent.dat
and param.dat
files. Assuming that you run the ADM1F: Execution time
example and produced the outputs_influent.csv
and outputs_params.csv
files, we use these outputs here to study the relationship between influents and outputs, and params and outputs. If not just uncomment lines 5 and 18 and
re-run the simulations.
Authors: Wenjuan Zhang and Elchin Jafarov
[1]:
import adm1f_utils as adm1fu
import os
import pandas as pd
import matplotlib.pyplot as plt
import seaborn as sns
%matplotlib inline
1. Influent/Output sensitivity¶
[2]:
# navigate to simulations folder
os.chdir('../../simulations')
[3]:
#Set the path to the ADM1F executable
ADM1F_EXE = '/Users/elchin/project/ADM1F_WM/build/adm1f'
# Set the value of percentage and sample size for lhs
percent = 0.1 # NOTE: for params percent should be <= 0.05
sample_size = 100
variable = 'influent' # influent/params/ic
method = 'uniform' #'uniform' or 'lhs'
[4]:
index=adm1fu.create_a_sample_matrix(variable,method,percent,sample_size)
print ()
print ('Number of elements participated in the sampling:',len(index))
Saves a sampling matrix [sample_size,array_size] into var_influent.csv
sample_size,array_size: (100, 11)
Each column of the matrix corresponds to a variable perturbed 100 times around its original value
var_influent.csv SAVED!
Number of elements participated in the sampling: 11
[5]:
#exe_time=adm1fu.adm1f_output_sampling(ADM1F_EXE,variable,index)
[6]:
[output_name,output_unit]=adm1fu.get_output_names()
alloutputs = pd.read_csv('outputs_influent.csv', sep=',', header=None)
alloutputs.columns = output_name
[7]:
alloutputs
[7]:
Ssu | Saa | Sfa | Sva | Sbu | Spro | Sac | Sh2 | Sch4 | Sic | ... | Alk | NH3 | NH4 | LCFA | percentch4 | energych4 | efficiency | VFA/ALK | ACN | sampleT | |
---|---|---|---|---|---|---|---|---|---|---|---|---|---|---|---|---|---|---|---|---|---|
0 | 7.37820 | 3.30795 | 56.7230 | 6.60272 | 8.46032 | 6.26942 | 1908.38 | 0.000123 | 48.7774 | 615.799 | ... | 8148.98 | 8.64671 | 987.952 | 56.7230 | 57.2720 | 66.2091 | 52.7615 | 0.222004 | 116.5620 | 24.3594 |
1 | 7.28460 | 3.26614 | 55.8953 | 6.52078 | 8.34621 | 6.18289 | 2033.37 | 0.000121 | 48.4068 | 645.849 | ... | 8602.48 | 9.61694 | 1048.750 | 55.8953 | 56.8728 | 65.9860 | 53.8907 | 0.223891 | 92.1431 | 24.8179 |
2 | 6.85866 | 3.07582 | 52.1661 | 6.11321 | 7.84990 | 5.79147 | 1926.30 | 0.000114 | 47.7048 | 651.060 | ... | 8442.25 | 9.57599 | 1031.740 | 52.1661 | 56.7510 | 61.6157 | 52.8110 | 0.216106 | 125.4410 | 27.1630 |
3 | 6.72726 | 3.01709 | 51.0289 | 5.97094 | 7.70738 | 5.67153 | 1931.76 | 0.000112 | 47.6768 | 660.852 | ... | 8558.31 | 9.84058 | 1044.000 | 51.0289 | 56.6698 | 61.9098 | 53.9655 | 0.213730 | 116.0810 | 27.9786 |
4 | 7.55763 | 3.38810 | 58.3212 | 6.83699 | 8.63179 | 6.43593 | 2242.26 | 0.000126 | 49.1049 | 657.957 | ... | 9061.95 | 10.49270 | 1109.360 | 58.3212 | 57.2318 | 68.8331 | 51.4620 | 0.234238 | 77.3666 | 23.5162 |
... | ... | ... | ... | ... | ... | ... | ... | ... | ... | ... | ... | ... | ... | ... | ... | ... | ... | ... | ... | ... | ... |
95 | 7.65859 | 3.43319 | 59.2237 | 6.78604 | 8.84032 | 6.52986 | 1911.45 | 0.000128 | 48.9244 | 616.258 | ... | 8197.36 | 8.37148 | 985.507 | 59.2237 | 56.5753 | 71.6467 | 52.7621 | 0.221140 | 125.5700 | 23.0752 |
96 | 7.40606 | 3.32040 | 56.9708 | 6.57190 | 8.52763 | 6.29525 | 2049.40 | 0.000123 | 48.6287 | 649.584 | ... | 8699.15 | 9.58421 | 1054.650 | 56.9708 | 56.5357 | 69.7230 | 54.2485 | 0.223168 | 102.1390 | 24.2222 |
97 | 7.06582 | 3.16840 | 53.9710 | 6.30301 | 8.09601 | 5.98132 | 1778.88 | 0.000118 | 48.2397 | 605.950 | ... | 7819.47 | 8.22490 | 949.405 | 53.9710 | 57.3804 | 61.6582 | 52.9718 | 0.215719 | 129.6520 | 25.9740 |
98 | 6.85456 | 3.07399 | 52.1312 | 6.12749 | 7.83407 | 5.78776 | 2146.27 | 0.000114 | 48.1629 | 685.484 | ... | 9144.20 | 11.07250 | 1119.840 | 52.1312 | 56.8556 | 64.3136 | 53.8121 | 0.222069 | 88.1808 | 27.1834 |
99 | 7.44704 | 3.33870 | 57.3346 | 6.67541 | 8.53678 | 6.33321 | 1983.08 | 0.000124 | 48.5798 | 627.253 | ... | 8349.28 | 9.04090 | 1016.750 | 57.3346 | 57.0513 | 66.1387 | 53.8114 | 0.225090 | 103.0960 | 24.0295 |
100 rows × 67 columns
[8]:
[influent_name,influent_index]=adm1fu.get_influent_names()
[9]:
# since we did not use all the columns in the influent.dat (see create_a_sample_matrix)
# we use index to select used headers for used values
header=[]
for i in index:
header.append(influent_name[i])
influent_inputs = pd.read_csv('var_influent.csv', sep=',', header=None)
influent_inputs.columns = header
influent_inputs.head()
[9]:
S_su_in | S_aa_in | S_fa_in | S_ac_in | S_IN_in | X_ch_biom_in | X_pr_biom_in | X_li_biom_in | X_I_in | Q | Temp | |
---|---|---|---|---|---|---|---|---|---|---|---|
0 | 2.41679 | 4.54049 | 3.26551 | 1.06715 | 0.00794 | 8.21103 | 7.65897 | 5.45471 | 18.04704 | 139.57635 | 31.64409 |
1 | 2.71197 | 4.44197 | 2.94117 | 0.97991 | 0.00798 | 8.47247 | 8.44312 | 5.01331 | 16.95071 | 136.99766 | 32.47646 |
2 | 2.37594 | 4.05372 | 3.09329 | 1.10619 | 0.00801 | 8.84280 | 8.55680 | 4.62146 | 18.06979 | 125.17005 | 31.95536 |
3 | 2.70155 | 4.55292 | 3.31319 | 1.00561 | 0.00784 | 9.14260 | 8.30096 | 4.69829 | 17.67225 | 121.52161 | 37.86524 |
4 | 2.35939 | 4.30043 | 3.00319 | 1.05070 | 0.00860 | 8.26193 | 9.19056 | 5.36216 | 19.24420 | 144.58137 | 35.68530 |
[10]:
# merge influent and output datasets
inout=pd.concat([influent_inputs,alloutputs], axis=1)
inout.head()
[10]:
S_su_in | S_aa_in | S_fa_in | S_ac_in | S_IN_in | X_ch_biom_in | X_pr_biom_in | X_li_biom_in | X_I_in | Q | ... | Alk | NH3 | NH4 | LCFA | percentch4 | energych4 | efficiency | VFA/ALK | ACN | sampleT | |
---|---|---|---|---|---|---|---|---|---|---|---|---|---|---|---|---|---|---|---|---|---|
0 | 2.41679 | 4.54049 | 3.26551 | 1.06715 | 0.00794 | 8.21103 | 7.65897 | 5.45471 | 18.04704 | 139.57635 | ... | 8148.98 | 8.64671 | 987.952 | 56.7230 | 57.2720 | 66.2091 | 52.7615 | 0.222004 | 116.5620 | 24.3594 |
1 | 2.71197 | 4.44197 | 2.94117 | 0.97991 | 0.00798 | 8.47247 | 8.44312 | 5.01331 | 16.95071 | 136.99766 | ... | 8602.48 | 9.61694 | 1048.750 | 55.8953 | 56.8728 | 65.9860 | 53.8907 | 0.223891 | 92.1431 | 24.8179 |
2 | 2.37594 | 4.05372 | 3.09329 | 1.10619 | 0.00801 | 8.84280 | 8.55680 | 4.62146 | 18.06979 | 125.17005 | ... | 8442.25 | 9.57599 | 1031.740 | 52.1661 | 56.7510 | 61.6157 | 52.8110 | 0.216106 | 125.4410 | 27.1630 |
3 | 2.70155 | 4.55292 | 3.31319 | 1.00561 | 0.00784 | 9.14260 | 8.30096 | 4.69829 | 17.67225 | 121.52161 | ... | 8558.31 | 9.84058 | 1044.000 | 51.0289 | 56.6698 | 61.9098 | 53.9655 | 0.213730 | 116.0810 | 27.9786 |
4 | 2.35939 | 4.30043 | 3.00319 | 1.05070 | 0.00860 | 8.26193 | 9.19056 | 5.36216 | 19.24420 | 144.58137 | ... | 9061.95 | 10.49270 | 1109.360 | 58.3212 | 57.2318 | 68.8331 | 51.4620 | 0.234238 | 77.3666 | 23.5162 |
5 rows × 78 columns
The correlation heat map matrix below shows that four influents have the highest impact on the results: X_ch_biom_in
, X_pr_biom_in
, X_li_biom_in
, and Q
.
[11]:
corr=inout.corr()
plt.figure(figsize=(21,5))
sns.heatmap(corr.iloc[0:11,11:-1], cmap=sns.diverging_palette(220, 10, as_cmap=True))
plt.title('Correlation Matrix [influent/results]',fontsize=16);
plt.ylabel('Influent',fontsize=16)
plt.xlabel('Outputs',fontsize=16)
[11]:
Text(0.5, 23.09375, 'Outputs')
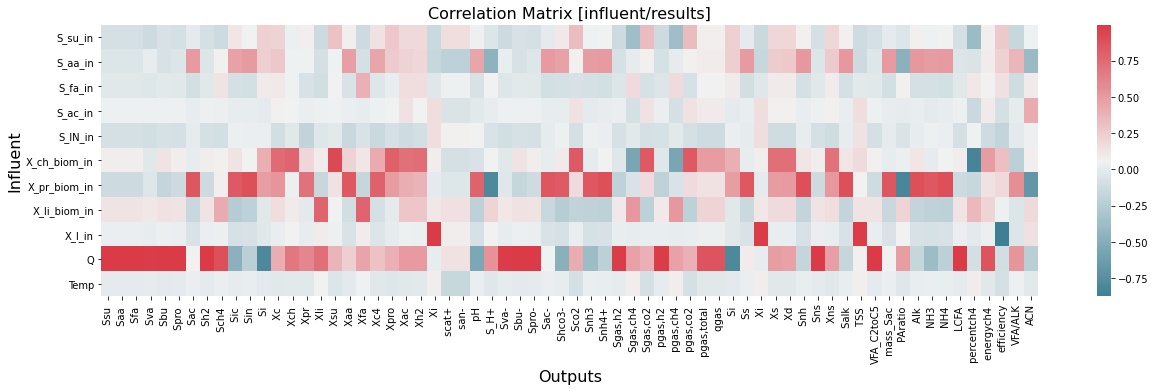
Let’s merge ph
values from the results with the influents and explore the correlations.
[12]:
influent_ph=pd.concat([influent_inputs,alloutputs[' pH ']], axis=1)
[13]:
plt.figure(figsize=(10,4))
influent_ph.corr().iloc[-1].plot(linewidth=2)
plt.title('Correlation plot (ph)')
plt.xlabel('Influents')
plt.ylabel('Correlation')
plt.ylim([-1,1])
[13]:
(-1.0, 1.0)
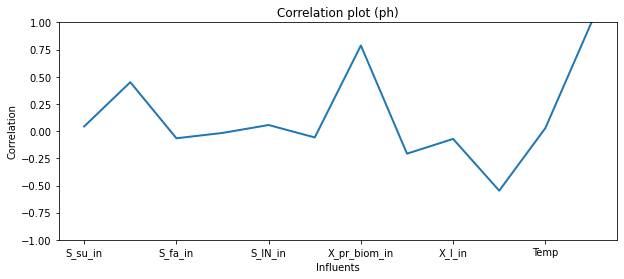
[14]:
plt.scatter( influent_ph['X_pr_biom_in'],influent_ph[' pH '])
plt.xlabel('X_pr_biom_in',fontsize=18)
plt.ylabel('pH',fontsize=18);
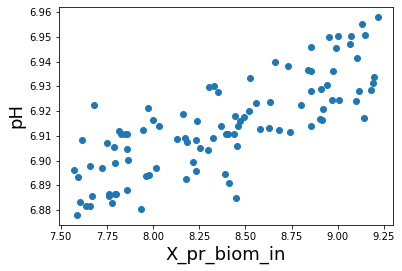
[15]:
from pandas.plotting import scatter_matrix
scatter_matrix(influent_ph, alpha=0.6,figsize=(10,10));
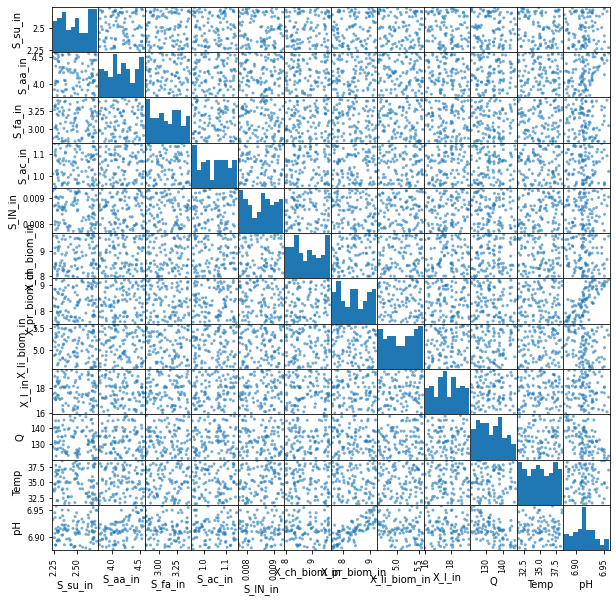
2. Params/Output sensitivity¶
[16]:
# Set the value of percentage and sample size for lhs
percent = 0.1 # NOTE: for params percent should be <= 0.05
sample_size = 100
variable = 'params' # influent/params/ic
method = 'uniform' #'uniform' or 'lhs'
[17]:
index=adm1fu.create_a_sample_matrix(variable,method,percent,sample_size)
print ()
print ('Number of elements participated in the sampling:',len(index))
Saves a sampling matrix [sample_size,array_size] into var_params.csv
sample_size,array_size: (100, 92)
Each column of the matrix corresponds to a variable perturbed 100 times around its original value
var_params.csv SAVED!
Number of elements participated in the sampling: 92
[18]:
#exe_time=adm1fu.adm1f_output_sampling(ADM1F_EXE,variable,index)
[19]:
[output_name,output_unit]=adm1fu.get_output_names()
alloutputs = pd.read_csv('outputs_params.csv', sep=',', header=None)
alloutputs.columns = output_name
[20]:
[param_name,param_index]=adm1fu.get_param_names()
[21]:
# since we did not use all the columns in the influent.dat (see create_a_sample_matrix)
# we use index to select used headers for used values
header=[]
for i in index:
header.append(param_name[i])
param_inputs = pd.read_csv('var_params.csv', sep=',', header=None)
param_inputs.columns = header
param_inputs.head()
[21]:
f_sI_xc | f_xI_xc | f_ch_xc | f_pr_xc | f_li_xc | N_xc | N_I | N_aa | C_xc | C_sI | ... | k_A_Bpro | k_A_Bac | k_A_Bco2 | k_A_BIN | kLa | K_H_h2o_base | K_H_co2_base | K_H_ch4_base | K_H_h2_base | k_P | |
---|---|---|---|---|---|---|---|---|---|---|---|---|---|---|---|---|---|---|---|---|---|
0 | 0.09116 | 0.27346 | 0.21535 | 0.21711 | 0.27475 | 0.00251 | 0.00420 | 0.00736 | 0.02895 | 0.02792 | ... | 1.026524e+10 | 1.086406e+10 | 9.205019e+09 | 1.087446e+10 | 207.51543 | 0.02859 | 0.03361 | 0.00146 | 0.00071 | 50821.70460 |
1 | 0.09692 | 0.25605 | 0.18183 | 0.21486 | 0.27367 | 0.00293 | 0.00450 | 0.00648 | 0.02930 | 0.02715 | ... | 9.961014e+09 | 9.209860e+09 | 9.484090e+09 | 1.097333e+10 | 185.69982 | 0.03129 | 0.03583 | 0.00146 | 0.00079 | 45097.70847 |
2 | 0.09653 | 0.25089 | 0.18351 | 0.19403 | 0.22666 | 0.00246 | 0.00420 | 0.00649 | 0.02824 | 0.03114 | ... | 1.043165e+10 | 1.081807e+10 | 9.359366e+09 | 9.475087e+09 | 218.85580 | 0.02930 | 0.03748 | 0.00140 | 0.00074 | 53707.49901 |
3 | 0.09891 | 0.25074 | 0.19437 | 0.20372 | 0.23318 | 0.00263 | 0.00468 | 0.00666 | 0.02873 | 0.02895 | ... | 9.431350e+09 | 1.031777e+10 | 9.787729e+09 | 1.030247e+10 | 184.26372 | 0.03229 | 0.03850 | 0.00127 | 0.00086 | 49069.07961 |
4 | 0.10742 | 0.26412 | 0.20268 | 0.20954 | 0.26893 | 0.00263 | 0.00414 | 0.00723 | 0.02958 | 0.03157 | ... | 1.090281e+10 | 1.020322e+10 | 1.063838e+10 | 1.076841e+10 | 189.12319 | 0.02950 | 0.03578 | 0.00138 | 0.00083 | 54000.23123 |
5 rows × 92 columns
[22]:
# merge influent and output datasets
inout=pd.concat([param_inputs,alloutputs], axis=1)
inout.head()
[22]:
f_sI_xc | f_xI_xc | f_ch_xc | f_pr_xc | f_li_xc | N_xc | N_I | N_aa | C_xc | C_sI | ... | Alk | NH3 | NH4 | LCFA | percentch4 | energych4 | efficiency | VFA/ALK | ACN | sampleT | |
---|---|---|---|---|---|---|---|---|---|---|---|---|---|---|---|---|---|---|---|---|---|
0 | 0.09116 | 0.27346 | 0.21535 | 0.21711 | 0.27475 | 0.00251 | 0.00420 | 0.00736 | 0.02895 | 0.02792 | ... | 8909.63 | 9.53919 | 1060.990 | 61.5561 | 56.0945 | 65.7436 | 52.8869 | 0.222452 | 87.1484 | 25.3731 |
1 | 0.09692 | 0.25605 | 0.18183 | 0.21486 | 0.27367 | 0.00293 | 0.00450 | 0.00648 | 0.02930 | 0.02715 | ... | 7707.69 | 10.88420 | 927.238 | 56.1139 | 62.1148 | 62.3272 | 55.0303 | 0.147138 | 654.5290 | 25.3731 |
2 | 0.09653 | 0.25089 | 0.18351 | 0.19403 | 0.22666 | 0.00246 | 0.00420 | 0.00649 | 0.02824 | 0.03114 | ... | 7873.10 | 8.67396 | 927.390 | 68.2248 | 58.8253 | 64.9817 | 54.1026 | 0.191081 | 196.3670 | 25.3731 |
3 | 0.09891 | 0.25074 | 0.19437 | 0.20372 | 0.23318 | 0.00263 | 0.00468 | 0.00666 | 0.02873 | 0.02895 | ... | 7961.45 | 10.83540 | 953.650 | 64.3854 | 57.4965 | 66.4942 | 55.2003 | 0.118815 | -1663.6400 | 25.3731 |
4 | 0.10742 | 0.26412 | 0.20268 | 0.20954 | 0.26893 | 0.00263 | 0.00414 | 0.00723 | 0.02958 | 0.03157 | ... | 8755.37 | 10.43910 | 1081.950 | 67.7978 | 56.9557 | 64.9350 | 53.1639 | 0.222974 | 94.6821 | 25.3731 |
5 rows × 159 columns
[23]:
corr=inout.corr()
plt.figure(figsize=(25,15))
sns.heatmap(corr.iloc[0:92,92:-1], cmap=sns.diverging_palette(220, 10, as_cmap=True))
plt.title('Correlation Matrix [params/results]',fontsize=16);
plt.ylabel('Parameters',fontsize=16)
plt.xlabel('Outputs',fontsize=16)
[23]:
Text(0.5, 113.09375, 'Outputs')
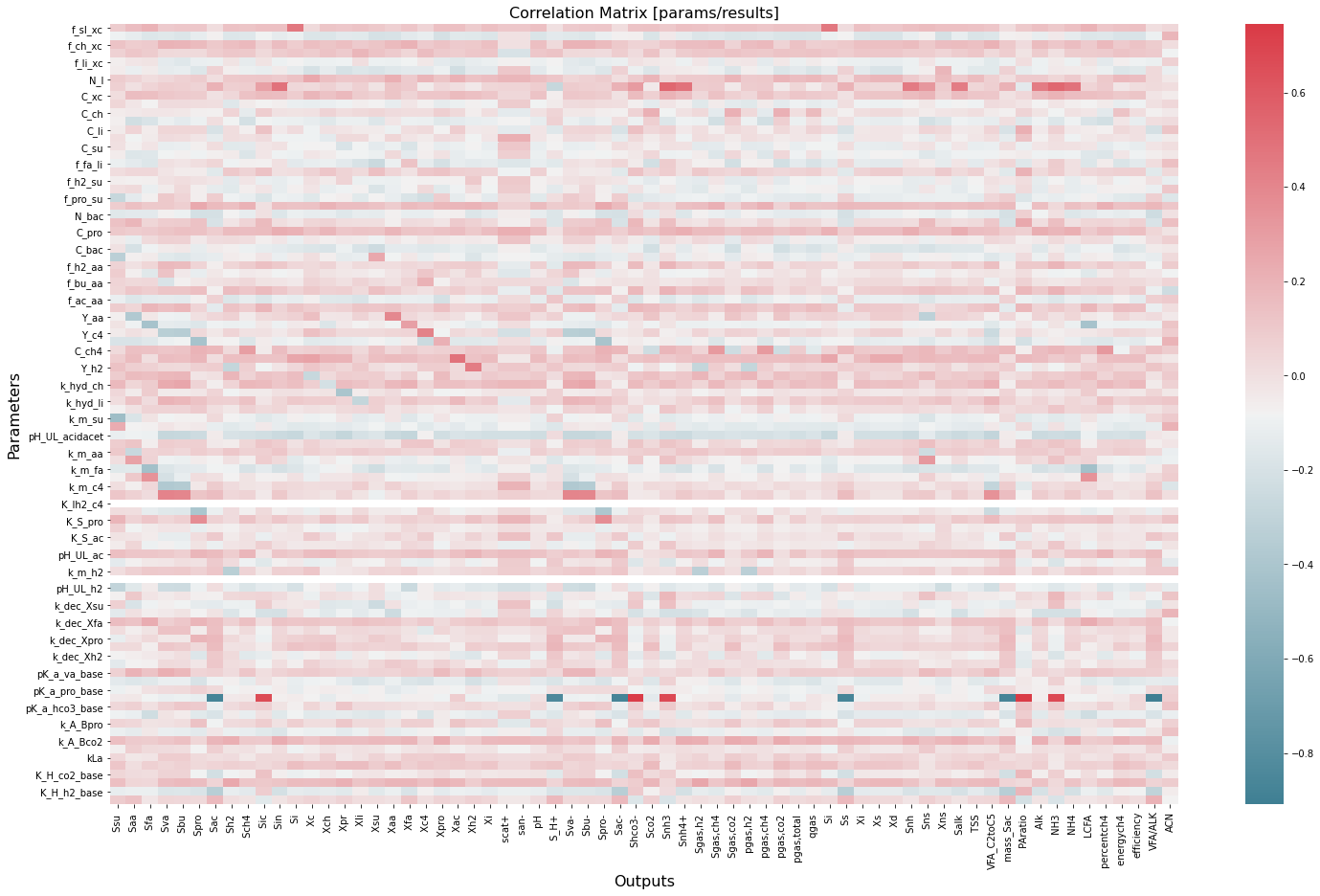
[ ]: